You know how much we love being at the forefront of technological reporting here at the QuickTSI Blog, and why not? The future of trucking is increasingly technological in nature. Whether it be using semi-autonomous technology, advanced safety systems, online training for new truck drivers, or otherwise, the trucking and transportation sector simply cannot escape it.
Did you know that the world generates 2.5 quintillion bytes of data every single day? That is a mind-boggling number! One of the sectors that will only further the rise of this number is transportation. Telematics and connected cars continue to be an increasing part of the sources that generate this massive onslaught of data. It is expected that by 2020, 10% of all data generated will come from vehicles that have embedded technologies.
By the same time, it is expected that there will be 152 million connected cars on the road and nearly 2 billion by 2025. One of the reasons for this massive increase in data is because of telematics. Telematics monitors both vehicles and drivers and collects a wealth of data, such as:
- Vehicle location
- Vehicle status
- Fuel usage
- Oil pressure
- Engine temperature
- Trip duration
- Odometer reading
- … and more
Yet, even though this seems like a huge amount of data, it is really just the tip of the iceberg. As vehicle technology continues to evolve, the types of data being collected will evolve with it. The question for fleets is: What do we do with all this data?
As we have talked about before, data is only useful if you know what to do with it. In fact, data for data’s sake can hamper a company, overloading the back office and confusing fleet managers. Motor carriers must utilize effective reporting to prioritize and analyze the data they are collecting.
How Reporting Impacts the Data
Telematics reporting has traditionally meant that a connected device collects vehicle and driver data then transmits it back to a data collection point. The fleet can set up specific categories that the data can be grouped into, whether it be the average speed of a vehicle or daily vehicle movements. Data can also be assigned to a specific truck driver and then sent on to the fleet manager for review.
Normally, a person will sift through and make sense of all that data. Unfortunately, this is a very time-consuming and error-prone way to sort through and evaluate large amounts of data. And even after the data has been put into specific categories, extra care needs to be put into retrieving the data when it is required.
Hand-managed data is also often quite goals-based. This means that all available data is collected and sent on to determine whether the fleet’s metrics are being met. This is also a scenario where it is up to the fleet manager to decide what data is applicable and not applicable, yet again putting them in a situation where simple human error could throw a wrench into the mix. With machine learning, however, that paradigm is changing.
Machine Learning Changes Data Reporting
We will eventually move to a system where it will not be all up to the fleet manager to collate and decide on what the right data is. Advances in artificial technology and machine learning will vastly improve how data is gathered and acted upon. The research processes behind machine learning and implementation are intimidating, but the concepts are quite simple. Even more, companies are stepping into the void to ensure traditional industries like trucking understand how to use these new, advanced tools.
You may be asking yourself: What is the difference between artificial intelligence and machine learning? It is helpful to consider that you cannot have one without the other. Machine learning is a core component of artificial intelligence. It is what gives the AI the ability to learn and improve from experience, which is also a critical component of human evolution. Machine learning allows an AI to act on new data without having to be programmed to do so.
AIs use machine learning to search for patterns in data, which then influences their future decisions. The more an AI analyzes and observes the data it is receiving the smarter it becomes. Now, take that concept and apply it to the manual job that fleet managers must do to ensure data integrity. Obviously, a machine could analyze the data, find patterns, and learn from it much faster.
Machine learning will also open new avenues in reporting and data management. Fleet managers will be able to focus on the most important data for the company’s needs. Motor carriers using these technologies will be able to see only the data that is most necessary for their needs. As the technology absorbs more data, metrics will need to be changed, but the overall data development cycle will continue.
How Machine Learning Will Impact Maintenance
One of the most critical aspects of operating an efficient fleet is ensuring the fleet is maintained. Predictive and preventative maintenance are at the core of those efforts. Technologies utilizing machine learning can measure precise changes in a vehicle’s engine and then notify an individual or designate a maintenance schedule. This can be done before a serious breakdown occurs.
Unexpected engine, or other technical failures, can cripple a fleet and seriously cut into the bottom line. With predictive analytics tied into machine learning, fleets can reduce downtime without seriously impacting manpower or established processes. Shop technicians will no longer have to rely solely on a schedule for maintenance. The problem with scheduled maintenance is that parts may be ordered that don’t end up being needed, which increases costs.
With integrated telematics incorporated with machine learning, maintenance can be completed when it is needed based on the parts available. With systems actively monitoring when a particular part is about to go out or is in need of active maintenance, the guesswork is removed from the equation.
Utilizing AI and machine learning for maintenance provides additional benefits, such as finding a remaining list of vehicles, determining maintenance “what if” scenarios. This will go even farther by allowing technicians to better maximize vehicle life by precisely handling maintenance needs on-the-spot.
All this is done through the ability to use the truck driver and vehicle to collect environmental data and combine it with historical facts, which allows for pattern recognition. This is called predictive analytics. And while predictive analytics does not definitively tell you what will happen in the future, it can accurately forecast future possibilities. The potential use for predictive data analytics is huge.
The Myriad Ways Predictive Analytics Helps Fleets
It isn’t just a maintenance failure that can cause serious cost problems for a fleet. Predictive analytics combined with machine learning can also help reduce overall fuel consumption. One example of how it does this is through monitoring tire pressure. When the tire pressure on a vehicle drops below a predetermined level, the shop can be notified.
AI-driven predictive analytics can also help determine what the best routes are. Whether it be to avoid high-traffic areas, construction, or roads or highways that are not properly maintained, all this helps to improve fuel efficiency and prevent waste. Even such factors as how much load is suitable for a particular vehicle or what behaviors truck drivers are exhibiting that waste fuel can have a big impact.
Machine learning can also help fleets paint a clearer picture of dangerous truck driver behaviors. Whether it be through excessive speeding or harsh acceleration, there is a risk posed to fleet safety when truck drivers don’t follow the rules of the road or the rules of the fleet.
Using the staggering amounts of data collected, motor carriers can predict future truck driver behavior. If truck drivers exhibited a certain behavior in the past, analytics allows fleet managers to give the appropriate level of training or corrective coaching. The goal is to improve how the truck driver operates the vehicle, rather than punitively punish them. One or two infractions does not make a truck driver a threat to fleet safety. Analyzing the data allows fleet managers to make the proper determination.
Consider that punishing a truck driver or sending them to training over something that may or may not be inherently their fault would be both ineffective and demotivating. Machine learning and predictive analytics can mitigate this potentially disastrous result.
The fact is this: We are entering a future where artificial intelligence and machine learning will open new avenues to addressing some of the most vexing problems fleets deal with. A greater variety of analytics and data processing tools only makes fleet stronger. As more companies move towards models that allow them to process data into actionable chunks faster and more efficiently, those not on the technology bandwagon will likely be left behind in a competitive marketplace. Will that be you?
from Quick Transport Solutions Trucking Blog https://ift.tt/2lefdlo


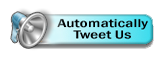
Sourced by Quik DMV - CADMV fleet registration services. Renew your registration online in only 10 minutes. No DMV visits, no lines, no phone mazes, and no appointments needed. Visit Quik, Click, Pay & Print your registration from home or any local print shop.
0 comments:
Post a Comment